Synthetic Data in AI (Part 2): The Essential Balance with Human-Curated Data
The future of synthetic data in AI, the risks of model collapse, and how innovations from the biggest names in the industry are shaping new best practices.

Aug 22, 2024
·
9
min read
Intro
In a previous edition, we explored synthetic data—artificially generated datasets that mimic real-world data—and its role in addressing data availability, privacy concerns, and bias in AI systems. As synthetic data grows in prominence, new developments and challenges have emerged that reshape our understanding of its potential and limitations. In this issue, we look further into the future of synthetic data and its role in the ongoing evolution of AI.
In this week's newsletter
What we’re talking about: The future of synthetic data in AI, the risks of model collapse, and how innovations from the biggest names in the industry are shaping new best practices.
How it’s relevant: As AI systems become more reliant on synthetic data, maintaining data quality is critical to avoid issues like "data pollution" and performance degradation. With advancements in data generation tools, organizations must balance innovation with the need for robust data management.
Why it matters: The responsible use of synthetic data will play a crucial role in shaping the accuracy and ethics of AI systems. Understanding these developments helps organizations stay ahead in AI innovation while avoiding potential pitfalls and regulatory challenges.
Big tech news of the week
🌏 A new GenAI weather model significantly improves the accuracy of weather predictions. This could help scientists take global climate change projections and more accurately apply them to local scales, and predict extreme weather such as floods and tornados.
🖥️ AMD buys ZT systems after completing an acquisition of Silo AI, an AI integration firm last week. The shopping spree is a key part of AMD’s strategy to narrow NVIDIA’s lead.
⚖️ SB 1047, a California bill aimed at regulating AI development, has recently gained attention due to its controversial nature and the responses from various stakeholders.
🚫 Elon Musk's social media platform, X, is facing significant legal challenges in Europe over allegations of unauthorized data use for training its AI model, Grok. In July X, formerly Twitter, quietly changed its data settings, automatically opting users in to train its new AI model on user data.
The Threat of Model Collapse
A looming issue has emerged in AI research: data pollution. Data pollution in AI refers to the presence of low-quality, inaccurate, biased, or irrelevant information in datasets used to train artificial intelligence models. This "polluted" data can negatively impact the performance, reliability, and fairness of AI systems.
As generative AI models become more reliant on synthetic data, they run the risk of producing outputs with decreasing variance and potentially replicating their own errors. This phenomenon, known as "model collapse," refers to the degradation of AI models that learn from data generated by other models.
In a paper published in Nature, researchers warn about the future of AI training data. Current AI models learn from a wide variety of human-created content - books, articles, websites, and social media posts. However, this pool of human-generated information isn't endless. By 2028, we might exhaust our supply of high-quality text data suitable for AI training. This could force the AI industry to rely heavily on synthetic data, creating a scenario where AIs primarily learn from other AIs. It's like making a photocopy of a photocopy - each generation loses some of the original's clarity and detail. The risk? A gradual loss of accuracy and diversity in AI outputs, as the subtle nuances and creativity of human expression get diluted with each iteration of AI-created content.
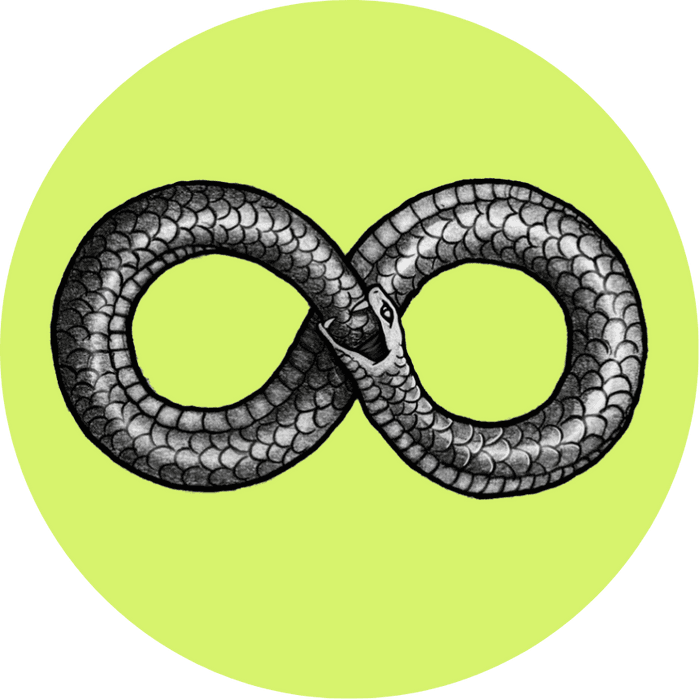
The risks of AI models "eating their own tails"—a metaphorical reference to the mythical serpent Ouroboros—may slow the rapid development of AI, as curating fresh, high-quality data becomes more difficult.
The Potential of Synthetic Data
Despite warnings of "data pollution," synthetic data continues to hold immense promise. Its privacy preservation, bias reduction, and potential to accelerate innovation make it a crucial tool for training AI models, particularly in sectors where real-world data is limited or inaccessible.
Companies like MOSTLY AI, a pioneer in the field of synthetic data since 2017, have played a significant role in proving the potential of AI-generated structured data. The company was among the first to release a Synthetic Data Platform. This platform addresses challenges posed by traditional data anonymization, which is the process of changing or removing personal information in a dataset so individuals can no longer be identified. Personal information can include obvious identifiers like names and addresses, but also combinations of data that could indirectly identify someone. Traditional anonymization methods often struggle to protect privacy while keeping the data useful for analysis, as simply removing names isn't always enough to prevent re-identification. These challenges became even more significant with the introduction of GDPR, which set stricter rules for handling personal data.

Innovative Approaches to Synthetic Data Generation
So what are the best practices when it comes to synthetic data? To ensure effective use, spending more time on data cleaning and validation and using synthetic data selectively is important. Precise generation of domain-specific data sets ensures higher quality and relevance in synthetic data outputs. New advancements like Meta’s Llama 3.1 and NVIDIA’s Omniverse Replicator are two examples that allow for this.
Llama 3.1 can create highly varied and nuanced instruction datasets, particularly in technical fields like coding or customer service dialogues. This control over the characteristics of synthetic data is particularly important in high-stakes industries such as medicine, where real-world data may be too sparse or sensitive to use. For example, in rare disease diagnosis, obtaining real patient data can be challenging due to the scarcity of cases and privacy concerns. In this case, using synthetic data could lead to improved diagnosing skills.
At the same time, platforms like NVIDIA's Omniverse Replicator use advanced tools to generate vast amounts of high-quality visual data for computer vision models. Imagine you need to simulate a rare scenario: an autonomous vehicle navigating through a blizzard at night. This kind of synthetic data can create such extreme conditions, allowing AI systems to learn from situations that are too dangerous or impractical to test in real life. With advanced GPUs (graphics processing units), Nvidia enables scalable synthetic data generation across systems designed for AI training and data analysis, reducing time and cost.
Addressing the Risks of Model Collapse and the Growing Importance of Human-Curated Data
As AI systems increasingly learn from the outputs of other models, there's a growing risk that synthetic data could degrade overall AI performance. This threat of model collapse underscores the need for careful management of synthetic data and highlights the enduring importance of human-curated data.
Industry leaders continue to develop advanced solutions to mitigate these risks. For instance, Nvidia's Nemotron-4, a family of advanced models, is specifically designed for generating high-quality synthetic data. These models utilize Reinforcement Learning with Human Feedback, learning from human preferences to generate outputs that are not only relevant but also aligned with the needs of specific industries, such as healthcare or finance.
It’s important to recognize that while synthetic data can help reduce biases present in real-world datasets, the algorithms used to generate synthetic data may inadvertently introduce new biases. Therefore, careful monitoring and validation of synthetic datasets are necessary to identify and mitigate these biases before using the data to train AI models.
Looking to the Future
As synthetic data becomes more widespread, high-quality human data will increase in value. This creates opportunities for businesses and researchers to focus on collecting fresh, private, and well-curated datasets, potentially gaining a "first-mover advantage" with models trained on unpolluted, human-generated data.
The ideal approach may be to combine synthetic and real-world data, creating hybrid datasets that optimize the strengths of both. Real-world data provides authentic variety, while synthetic data can fill gaps by generating underrepresented scenarios or attributes.
Looking ahead, as synthetic data use grows, regulatory bodies may introduce new guidelines or requirements, particularly in sensitive domains like healthcare and finance. Organizations leveraging synthetic data must stay informed about these emerging regulations to ensure compliance and maintain ethical AI practices.
Lumiera offers tailored strategies, usage guidelines, and business policies to help organizations remain compliant in their AI decisions.
Get free weekly insights straight to your inbox
No spam, unsubscribe at any time